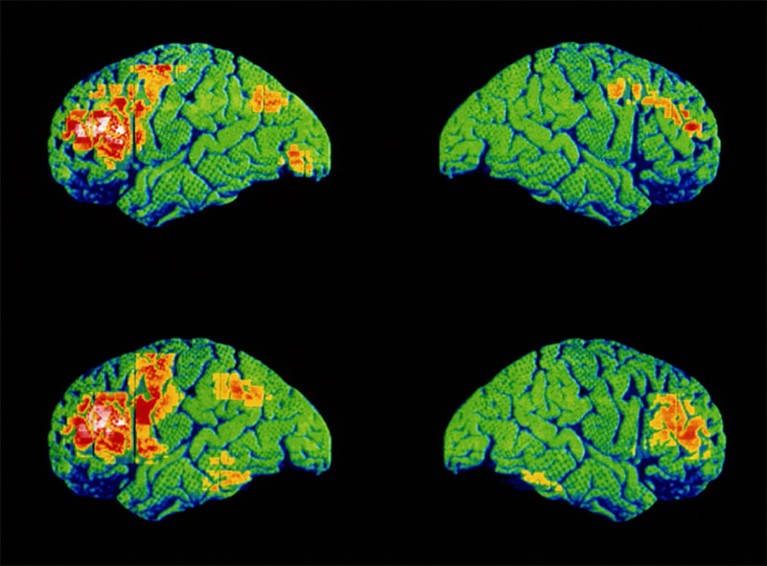
Computer algorithms that are designed to help doctors treat people with schizophrenia do not adapt well to fresh, unseen data, a study has found.
Such tools — which use artificial intelligence (AI) to spot patterns in large data sets and predict how individuals will respond to a particular treatment — are central to precision medicine, in which health-care professionals try to tailor treatment to each person. In work published on 11 January in Science1, researchers showed that AI models can predict treatment outcomes with high accuracy for people in a sample that they were trained on. But their performance drops to little better than chance when applied to subsets of the initial sample, or to different data sets.
To be effective, prediction models need to be consistently accurate across different cases, with minimal bias or random outcomes.
“It’s a huge problem that people have not woken up to,” says study co-author Adam Chekroud, a psychiatrist at Yale University in New Haven, Connecticut. “This study basically gives the proof that algorithms need to be tested on multiple samples.”
Algorithm accuracy
The researchers assessed an algorithm that is commonly used in psychiatric-prediction models. They used data from five clinical trials of antipsychotic drugs, involving 1,513 participants across North America, Asia, Europe and Africa, who had been diagnosed with schizophrenia. The trials, which were carried out between 2004 and 2009, measured participants’ symptoms before and four weeks after taking one of three antipsychotic drugs (or compared the effects of different doses of the same drug).
The team trained the algorithm to predict improvements in symptoms over four weeks of antipsychotic treatment. First, the researchers tested the algorithm’s accuracy in the trials in which it had been developed — comparing its predictions with the actual outcomes recorded in the trials — and found that the accuracy was high.
Then they used several approaches to evaluate how well the model generalizes to new data. The researchers trained it on a subset of data from one clinical trial and then applied it to another subset from the same trial. They also trained the algorithm on all the data from one trial — or a group of trials — and then measured its performance on a separate trial.
The model performed poorly in these tests, generating seemingly almost random predictions when applied to a data set that it had not been trained on. The team repeated the experiment using a different prediction algorithm, but got similar results.
Better testing
The study’s authors say that their findings highlight how clinical prediction models should be tested rigorously on large data sets to ensure that they are reliable. A systematic review2 of 308 clinical-prediction models for psychiatric outcomes found that only about 20% of models underwent validation on samples other than the ones on which they were developed.
“We should think about it much more like drug development,” says Chekroud. Many drugs show promise in early clinical trials, but falter in the later stages, he explains. “We do have to be really disciplined about how we build these algorithms and how we test them. We can’t just do it once and think it’s real.”