Day: 01/06/2024
Tech in a time of crisis
Does smartphone surveillance have a role to play in the fight against the coronavirus pandemic?
The 6G Wireless Summit was scheduled to take place in Levi, Finland on Tuesday 17 March. Five days before, the event was cancelled: 17 students at the University of Oulu, the institution organizing the summit, had been diagnosed with COVID-19. Given the wider context of the ongoing coronavirus pandemic, the cancellation was a minor inconvenience for the researchers in academia and industry that were planning to come together to discuss the future of wireless communications technology. And thanks to the resourcefulness of the organisers, the event was quickly retooled as a virtual event, with online presentations, Q&A sessions, and discussion forums.
Back in January, we highlighted the potential of the latest generation of wireless communications technology — 5G — and our exploration of the topic continues in this issue of Nature Electronics. In a Comment article, Shuangfeng Han and colleagues at the China Mobile Research Institute argue that 5G has an energy problem: the 5G era is expected to lead to a substantial increase in the power consumption and carbon emissions of wireless communication networks. And thus, developing strategies to optimize energy efficiency must be a priority for the industry.
Communications technology has taken on a renewed significance in recent weeks — labs and offices closed, social distancing practices imposed, working from home where possible — and is helping friends, families and colleagues to stay connected. But is there another role for the technology in the fight against the coronavirus pandemic?
In various locations around the world, smartphones are being used to try to control the spread of the virus. In South Korea, authorities have been sending text messages to residents detailing the movements (as well as personal details like age and gender) of local people who have recently tested positive1. In China, phone apps have been deployed that provide a colour-coded health designation (green, free movement; yellow, seven-day quarantine; red, two-week quarantine) and QR code; the codes are based on a person’s location, health details and travel history, and can limit where they can go2,3. In Taiwan, phone tracking is being used to help enforce quarantines4. And in Israel, data from mobile phones is being used to track the movements of people who have contracted the virus and to notify anyone who may have come into contact with them5.
There have been calls in the United States6 and across Europe7,8 to use data from mobile phones in similar efforts to restrain the coronavirus outbreak, and various relevant apps have already emerged. A team led by Ramesh Raskar at the Massachusetts Institute of Technology has, for example, created an app called Private Kit, which stores 28 days of location data that a user can choose to share with health officials if they become infected; a forthcoming update could allow the app to notify users if they have been in close contact with anyone diagnosed with the disease. Alternatively, a European initiative called Pan-European Privacy-Preserving Proximity Tracing has announced that it is working on using Bluetooth technology to record when a smartphone is near another device (information that can subsequently be used to track down people that have been near someone who has tested positive) in an approach that would be in line with the European Union’s General Data Protection Regulation (GDPR)9.
Recent work from researchers at the University of Oxford also highlights the potential importance of such technology10. Based on a mathematical analysis of transmission, they show that traditional manual contact tracing — in which a patient is asked to recall their movements and then anyone that they have recently been in contact with is tracked down and quarantined — is too slow to contain the spread of the virus; a contact-tracing app could though control the epidemic, if used by enough people. The researchers also suggest that the app could help at any stage of the epidemic: when it is just emerging, when it is at its peak, and when a region is trying to transition out of a widespread lockdown.
Smartphone surveillance does, however, bring with it ethical concerns. What data is shared and with whom? And if citizens are happy to grant governments access to information now, in a time of crisis, is there any guarantee that access will be relinquished once it is over? If the value of such technology is clear, it still needs to be implemented carefully.
Shining a light on COVID-19
Research activities in the areas of X-ray imaging and ultraviolet sterilization illustrate how photonics is helping to combat the threat of COVID-19.
As the COVID-19 pandemic caused by the SARS-CoV-2 coronavirus sweeps around the globe causing widespread misery and disruption, photonics researchers are considering how they can help. At the virtual IEEE International Symposium on Biomedical Imaging (ISBI 2020; http://2020.biomedicalimaging.org/) in early April, a special online session entitled ‘COVID-19, deep learning and biomedical imaging’ brought together a panel of six imaging experts from the US, Israel, the Netherlands and Denmark to discuss opportunities. The debate centred on how artificial intelligence (AI) could perhaps help by performing automated analysis and classification of chest X-rays and computer tomography (CT) scans from the lungs of patients with COVID-19, making it possible to identify the presence of the disease and assess its severity.
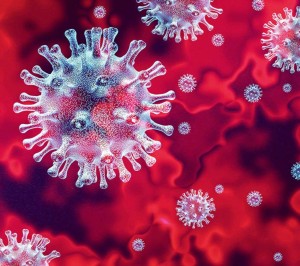
Credit: Brain light / Alamy Stock Photo
The idea and hope is that well-trained algorithms applied to lung images may provide an automated clinical means of diagnosis of COVID-19 that has a high degree of confidence and does not require any reagents, disposable consumables or lengthy processing times unlike swab polymerase chain reaction (PCR) tests. It is now known that the pneumonia associated with COVID-19 tends to present several typical signatures in lung images — in particular, so-called reverse halos, crazy-paving and ground glass features. Using AI to identify COVID-19 thus seems to be a realistic proposition.
“Chest radiographs cost under US$1 but their interpretation is difficult,” Eliot Siegel, chief of radiology at the University of Maryland School of Medicine in the US told attendees. “Can we use AI to distinguish COVID-19 from other respiratory diseases? Can we quantify the disease? Can we distinguish between acute and mild versions and track its trajectory?”
Bram van Ginneken from the diagnostic image analysis group at Radboud University in the Netherlands described efforts in the country to collect and analyse CT images of patients with COVID-19, yielding a standardized classification and diagnosis scheme called CORADS that has a score of one to six, with four and above indicating a high level of suspicion of COVID-19 infection (https://radiologyassistant.nl/chest/covid-19-corads-classification).
“There’s an enormous shortage of PCR swab tests, you have to wait for results and if the infection has moved from the nose and throat to the lungs it may not be detected by swab tests,” said van Ginneken. “Eight radiologists in seven Dutch hospitals have been assessing COVID-19 cases and we’ve found that the CORADS clinical diagnosis and the PCR test agree well.”
Furthermore, researchers say that AI may also be able to judge the severity of the disease, providing a quantitative score or metric that ranks the patient’s condition and prognosis, opening the way for new forms of triage and screening at hospitals.
Hayit Greenspan from Tel-Aviv University described efforts to develop a ‘Corona-Score’ that provides a quantitative metric for patients with COVID-19 by determining the volume of opacities in their lungs by applying algorithms to a three-dimensional fusion of image slices. “The Corona-Score represents the extent of the disease and strongly correlates to the severity of the disease,” explained Greenspan.
In a similar vein, in the US, Raul San Jose Estepar from Harvard University described the team’s development of an app called Covictory (details at https://acil-bwh.github.io/slowdown-covid19/). The idea is that a smartphone is used to take a photo of a portable chest X-ray image and the app uses AI algorithms to determine if the image likely represents normal healthy condition or mild or moderate/severe COVID-19, providing percentages for each situation. The thinking is that such a system may be a useful additional screening tool.
Moving away from the discussion at ISBI, scientists and tech firms are exploring the opportunities for ultraviolet (UV) sterilization as a convenient means for eradicating coronavirus from pieces of personal protective equipment (PPE) and contaminated surfaces. It’s well established that short-wavelength UV-C light (200–280 nm region) is highly effective at killing bacteria and viruses by damaging their genetic material (DNA or RNA) if the dose of the light is sufficient, thus providing an environmentally friendly, chemical-free means for sterilization.
With this in mind, Andrea Armani’s group from the University of Southern California has developed a simple UV-C chamber for sterilizing face visors. The system consists of 18-gallon plastic bins that are internally coated with highly reflective chrome paint and equipped with 15-W high-intensity UV-C bulbs. Armani says that a chamber costs just US$50, can hold 3 face shields at once, and it takes 6 minutes to perform a sterilization cycle. By working with a local firm in Los Angeles, 50 systems have been manufactured and donated to local hospitals in the region. So far, the team has focused on sterilization of face shields as this is straightforward and works well, and Armani says that other pieces of equipment such as N95 masks, “could be tricky” due to their more complex composition. Interestingly, the Danish firm UVD Robots has taken things a stage further and has equipped autonomous robots with UV light sources in order to perform automated sterilization of spaces. It takes about 10–20 minutes for its disinfection robot to treat a room, according to the firm.
It is likely that the valuable contributions from vaccine hunters, epidemiologists and virologists will be best remembered for how science ultimately triumphed over COVID-19, but it’s possible that photonics may play a small role along the way.
Nanotechnology-based disinfectants and sensors for SARS-CoV-2
Nanotechnology-based antimicrobial and antiviral formulations can prevent SARS-CoV-2 viral dissemination, and highly sensitive biosensors and detection platforms may contribute to the detection and diagnosis of COVID-19.
One thing we have learned so far amid the current coronavirus disease 2019 (COVID-19) pandemic is the degree to which we are limited in our fight against respiratory viral diseases. Up to now SARS-CoV-2 has spread to over 215 countries, with more than 15,000,000 people infected, and over 615,000 deaths to date (Johns Hopkins University Coronavirus Resource Center, 21 July 2020). Our most important line of defence is our own immune system, however people who are immunocompromized, or people with at least one underlying co-morbidity (that is, cardiovascular diseases/hypertension and diabetes, and other chronic underlying conditions), are highly vulnerable and their sole line of defence is sanitizers, face masks, immune system boosters and drugs that are clinically approved1. Scientists around the world have made promising strides towards developing approaches to prevent COVID-192. However, there are still challenges for the development of therapeutics or vaccines, such as regulatory issues, large-scale production and deployment to the public3. It will take months before we can have a global answer to this pandemic. Furthermore, we must be prepared for potential outbreak of a second and even a third wave of the virus, which calls for alternative options to reinforce our arsenal against not only COVID-19 but also other viral diseases that can potentially become pandemics. The silver lining amidst this crisis is the state of our technological advances mainly in the field of nanotechnology. So far, a significant body of work has covered the development of nano-based vaccines or anti-viral agents to block SARS-CoV-2, all of which are currently far from public implementation due to lengthy and strict regulatory affairs4.
Consequently, we propose that nanotechnology could have a closer impact on the current pandemic when implemented in two defined areas: (1) Viral disinfectants, by developing highly effective nano-based antimicrobial and antiviral formulations that are not only suitable for disinfecting air and surfaces, but are also effective in reinforcing personal protective equipment such as facial respirators. (2) Viral detection, by developing highly sensitive and accurate nano-based sensors that allow early diagnosis of COVID-19.
Viral disinfectants
Considering various transmission routes of coronavirus (that is, via cough or respiratory droplets, or biofluids)5, one approach to fight against the virus is through preventing its dissemination by means of disinfecting air, skin or surrounding surfaces (Fig. 1).
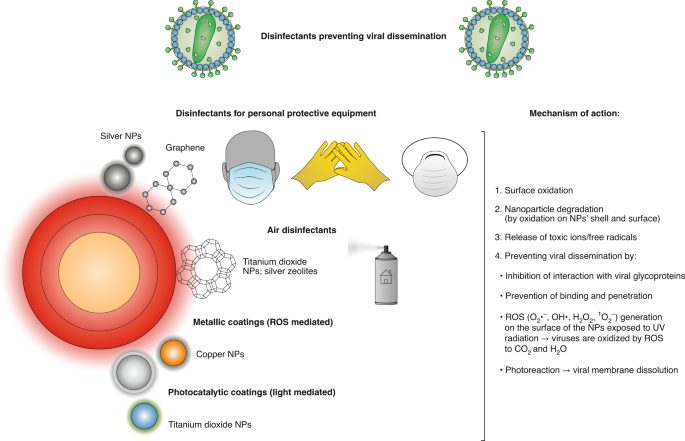
To this end, chemical disinfectants (such as chlorines, peroxides, quaternary amines and alcohols) effective against a wide variety of pathogens have been used for disinfection and sterilization of personal protective equipment and surfaces6. Despite promising results from chemical disinfectants, they are often associated with drawbacks such as high concentration requirements for 100% viral inhibition, limited effectiveness over time, and possible risks to public health and environment7,8. Consequently, metallic nanoparticles (for example, silver, copper, titanium dioxide nanoparticles) have been proposed as alternatives due to their inherent broad range antiviral activities, persistence and ability to be effective at much lower dosage9,10. For instance, preliminary evaluations showed that silver nanocluster/silica composite coating on facial masks had viricidal effects against SARS-CoV-211. In another example, NanoTechSurface, Italy, developed a durable and self-sterilizing formula comprised of titanium dioxide and silver ions for disinfecting surfaces12. In a similar manner, FN Nano Inc., USA, developed a photocatalytic coating (light mediated) based on titanium dioxide nanoparticles, which can decompose organic compounds including viruses on the surface upon exposure to light, damaging the viral membrane12. Nanomaterials can also be incorporated into respiratory face masks to further increase their inhibitory effect13. Scientists from Queensland University of Technology, Australia, have developed a breathable and disposable filter cartridge from cellulose nanofibers, which were capable of filtering particles smaller than 100 nanometres14. Alternatively, owing to high surface-area-to-volume ratio and their unique chemical and physical properties, other nanomaterials (for example, graphene) can be used to adsorb and eliminate SARS-CoV-215. For instance, LIGC Applications Ltd., USA, have made a reusable mask made of microporous conductive graphene foam that allows the trapping of microorganisms and the conduction of electrical charge to destroy them16.
These nanomaterials present an enormous potential as disinfectants against coronavirus, mainly due to unique attributes of nanomaterials including intrinsic anti-viral properties such as reactive oxygen species (ROS) generation and photo-dynamic and photo-thermal capabilities. Also, adverse effects of metallic nanomaterials on human health and the environment can be prevented by using biodegradable nanomaterials (that is, polymeric, lipid-based).
Viral detection
Diagnostics is a critical weapon in the fight against this pandemic, as it is pivotal to isolate infected individuals as early as possible, preventing dissemination17. Several nanotechnology-based approaches for SARS-CoV-2 tagging and detection are being developed (Fig. 2).
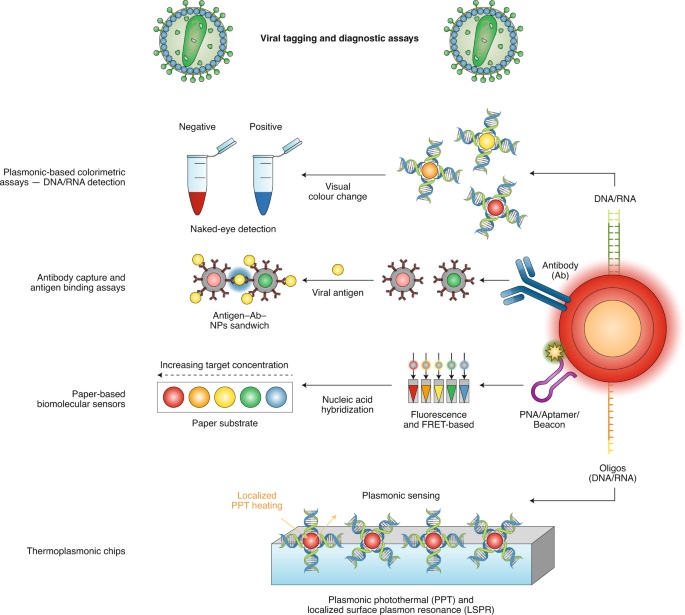
Generally, testing kits operate based on detection of antibodies (by enzyme-linked immunosorbent assay, or enzyme-linked immunosorbent assay (ELISA)) or RNA (by polymerase chain reaction, or PCR) associated with the virus (from nasopharyngeal swabs taken from individuals’ noses and throats). This relies on their surface interactions with a complementary detection ligand or strand in the kit18. However, these testing kits are generally associated with problems such as false-negative results, long response times and poor analytical sensitivity19. To this end, due to their extremely large surface-to-volume ratios, nanosized materials can instigate highly efficient surface interactions between the sensor and the analyte, allowing faster and more reliable detection of the virus20. Accordingly, a group of researchers have developed a colloidal gold-based test kit that enables easy conjugation of gold nanoparticles to IgM/IgG antibodies in human serum, plasma and whole blood samples21. However, the targeted IgM/IgG antibodies in this kit were not specific to COVID-19, and as a result in some cases produced false results associated with patients who were suffering from irrelevant infections. Consequently, researchers from the University of Maryland, USA, developed a colorimetric assay based on gold nanoparticles capped with suitably designed thiol-modified DNA antisense oligonucleotides specific for N-gene (nucleocapsid phosphoprotein) of SARS-CoV-2, which were used for diagnosing positive COVID-19 cases within 10 min from the isolated RNA samples22. Such testing kits could potentially produce promising results, however their performance would still be affected by quantity of the viral load. To address this shortcoming, researchers from ETH, Switzerland, have recently reported a unique dual-functional plasmonic biosensor combining the plasmonic photothermal effect and localized surface plasmon resonance (LSPR) sensing transduction to provide an alternative and promising solution for clinical COVID-19 diagnosis23. The two-dimensional gold nano-islands functionalized with complementary DNA receptors provide highly sensitive detection of the selected sequences from SARS-CoV-2 through nucleic acid hybridization. For better sensing performance, thermoplasmonic heat is generated on the same gold nano-islands chip when illuminated at their plasmonic resonance frequency. Remarkably, this dual-functional LSPR biosensor exhibited high selectivity towards the SARS-CoV-2 sequences with a detection limit as low as 0.22 pM. In other work, to achieve rapid and accurate detection of SARS-CoV-2 in clinical samples, researchers from the Korea Basic Science Institute developed an ultra-sensitive field-effect transistor (FET)-based biosensing device24. The sensor was produced by coating graphene sheets of the FET with a specific antibody against SARS-CoV-2 spike protein. The FET device could detect the SARS-CoV-2 spike protein at concentrations of 1.31×10–5 pM in phosphate-buffered saline and 1.31×10–3 pM in clinical transport medium. Remarkably, the device exhibited no measurable cross-reactivity with Middle East respiratory syndrome coronavirus (MERS-CoV) antigen, indicating the extraordinary capability of this sensor to distinguish the SARS-CoV-2 antigen protein from those of MERS-CoV.
Another approach that can be used for SARS-CoV-2 and that was successfully used with MERS-CoV, Mycobacterium tuberculosis and human papillomavirus consists of a paper-based colorimetric sensor for DNA detection based on pyrrolidinyl peptide nucleic acid (acpcPNA)-induced silver nanoparticle aggregation25. Briefly, in the absence of complementary DNA, silver nanoparticles aggregate due their electrostatic interactions with the acpcPNA probe. However, in the presence of target DNA, a DNA–acpcPNA duplex starts to form which leads to dispersion of the silver nanoparticles as a result of electrostatic repulsion, giving rise to a detectable colour change25. The use of aptamers and molecular beacons instead of PNA can also represent a potential alternative.
Other avenue where nanomaterials can contribute to detection of SARS-CoV-2 is the extraction and purification of targeted molecules from biological fluids (blood and nasal/throat samples). Thus, nanomaterials with magnetic properties can be decorated with specific receptors of the virus, leading to attachment of virus molecules to the nanoparticles that will allow their magnetic extraction using an external magnetic field.
In this way nanomaterial-based detection can facilitate faster and more accurate detection of the virus even at early stages of the infection, in large due to versatility of surface modification of nanoparticles.
Outlook
This overview of newly developed nanotechnology-based disinfectants and sensors for SARS-CoV-2 lays out a blueprint for development of more effective sensors and disinfectants that can be implemented for the purpose of detection, and prevention of this and another coronavirus. More advances in nano-based disinfectants are needed to meet the challenges on the front lines of patient care. On the other side, with COVID-19 rapidly spreading and with new foci of infection around the corner, efficient detection is pivotal, and the rule is to diagnose more quickly, easily and broadly. Time is of essence when dealing with pandemics and the two emphasized aspects of nanotechnology are more likely to soon become available to the public, as they are not associated with some of the stricter regulations commonly associated with vaccines. It is essential to shorten patient-specific and community-wide response times to determine who is infected or not and nanotechnology products like the ones described here will also reduce the impact on healthcare workers by providing faster and easy-to-use platforms that do not require special equipment or highly trained personnel. And this is how nanotechnology is taking root against SARS-CoV-2, by promoting exactly the type of wide-ranging, integrated approaches that are essential to control this pandemic outbreak at local, national, and international levels.
Machine Learning for COVID-19 needs global collaboration and data-sharing
The COVID-19 pandemic poses a historical challenge to society. The profusion of data requires machine learning to improve and accelerate COVID-19 diagnosis, prognosis and treatment. However, a global and open approach is necessary to avoid pitfalls in these applications.
On 31 December 2019, the first cases of a viral pneumonia with unknown aetiology were reported in the city of Wuhan, China. In the following weeks, the Chinese authorities and the World Health Organization (WHO) announced the discovery of a novel coronavirus and its associated disease: SARS-CoV-2 and COVID-19, respectively. On 21 April 2020, the number of cases of COVID-19 exceeded 2.4 million and the death toll exceeded 170,000 worldwide1. The outbreak of COVID-19 represents a major and urgent threat to global health. While the unprecedented speed of the COVID-19 spread partly finds its roots in our increasingly globalized society, the global sharing of scientific data also offers a promising tool to fight the disease. In the past four months, more than 12,400 articles have been published2 and scientific data collected from thousands of patients have been released3. The majority of these studies follow the standard scientific method: that is, investigate a few hypotheses at a time on a controlled sample. While undeniably successful, this standard method suffers from two well-known challenges, both critical to our pandemic situation: (1) it requires considerable expertise and human input and (2) it only considers a handful hypotheses at a time. Machine learning (ML) has been used to meet these challenges in various pathologies4,5, including infectious diseases6. Herein, we describe two areas where ML could supplement standard statistical methods in the COVID-19 pandemic, discuss the practical challenges that such a ML approach entails, and advocate for a global collaboration and data-sharing.
ML to alleviate the workload of medical experts
While standard statistical methods can provide the first results necessary in an emergency, they often require considerable human resources, which are precisely lacking in such context. Health systems found themselves quickly overwhelmed and the potential for data analysis, particularly in clinical research, was limited by the amount of work required. ML techniques can decrease the time required to produce automated analyses and allow artificial intelligence practitioners to support clinicians. For example, medical imaging studies show that chest computed tomography (CT) scans can be used to detect COVID-19 lesions7,8. However, such studies typically require each scan to be reviewed by a trained radiologist, who could otherwise be working on the front lines. ML may alleviate this task: recent supervised classifiers trained over a large dataset of 400,000 chest X-Rays achieved a mean area under the receiver operating characteristic curve (false positive rate versus true positive rate) of 94% for the diagnosis of 14 distinct lung pathologies9. Furthermore, preliminary studies based on a few hundred chest CT scans suggest that COVID-19 can be automatically diagnosed with ML10. However, the use of ML of medical images to diagnose or prognose COVID-19 remains currently limited to relatively small cohorts. These studies thus poorly control for the numerous confounds (for example, age, corpulence) that the algorithms may detect from chest images. A promising strategy is to pre-train ML models from larger datasets of similar images, thus learning common features to compute, which can then be used to facilitate training from COVID-19 images. This strategy has been used again and again in computer vision in recent years, to achieve impressive results in tasks with few labelled examples11.
ML to accelerate the screening of treatments
Standard methods only consider a handful hypotheses at a time. For example, among more than 1,200 clinical trials that have been registered to identify treatments for COVID-19, the majority focus on a unique drug or a couple of drugs, hand-selected on rationales of varying relevance12. ML can broaden such a screening and selection process by simultaneously considering several potential antiviral agents, relying on DNA sequences and/or protein structure, including potential drug binding sites of SARS-CoV-2, to predict interactions between drugs and the virus, and thus shortlisting promising candidate treatments13,14. ML has been used in other infectious diseases in a similar fashion15: for example, a deep neural network was successfully trained to screen the activity of more than 100 million molecules on Escherichia coli16. In the same way, a large spectrum of vaccine candidates could be screened based on their potential to elicit an effective immune response, for example, by presenting the spike protein S that follows a SARS-CoV-2 infection17. Nonetheless, these potentially fruitful avenues should not hide the challenges of therapeutic research based on ML. First, ML cannot accelerate basic biology, and even the prediction of protein folding remains a remarkably difficult problem18. In the case of vaccines, there is therefore a necessary waiting period. Second, a major ethical concern is the temptation to bypass proper clinical trials: working with very small cohorts, not using adequate design, or omitting inclusion and exclusion criteria have already be reported in the recent hydroxychloroquine-based treatment research19. This risk could dramatically increase with ML algorithms. Indeed, algorithms such as deep neural networks are ‘general approximators’: they can be trained to fit any objective on a dataset by, for example, memorizing the diagnosis of every patients. ML algorithms can only be evaluated conclusively by assessing their ability to accurately predict an independent test set — an approach that necessitate large datasets and a priori inclusion and exclusion criteria.
A major need for data sharing
While standard statistical analyses are adapted for many clinical and epidemiological challenges, ML is essential to accelerate the analysis of complex and large datasets such as large genomic or medical imaging datasets. Overall, ML is thus promised to supplement rather than supersede standard methods used for diagnoses, prognosis and treatment. However, two major challenges currently limit the potential impact of ML. First, ML algorithms are notoriously difficult to interpret. While visualization tools may highlight the combination of variables that led an algorithm to make a particular prediction, healthcare professionals must be aware that, like humans, ML can easily be affected by systematic biases (for example, scanning device, patient’s age and so on). Special pedagogical efforts must thus be made in both scientific reports and in the clinics to maintain a healthy scepticism when it comes to ML findings. Second, the lack of large healthcare, clinical, imaging and genetic public repositories leads each institution to locally develop its own analytical pipeline on its own small dataset, which significantly limits the generalizability of the results. While this issue is not specific to ML, the ability of modern algorithms to encompass heterogeneous datasets should drive us to both (1) share the de-anonymized raw data used in each clinical study, and (2) favour the development of large cohorts. The International Severe Acute Respiratory and emerging Infection Consortium (ISARIC) initiative aims to provide a large and shared clinical database on COVID-19 patients20. Other institutions have signed data-sharing agreements to ensure that data is shared widely and rapidly21,22, and can inform new hypotheses, but this is still done in a piecemeal fashion, making it difficult to make the most of the data generated daily during the pandemic. Not only will the quality of the standard and ML models directly depend on the size, quality and representativeness of such databases, but they will be critical to support effective interventions across different countries and types of healthcare facilities6. Open sharing of clinical databases requires significant care to properly manage regulatory and data privacy issues. Rapidly resolving these issues can be particularly challenging during a pandemic, when many public institutions are not operating normally. However, until we meet these challenges, ML may not keep its promises to help fight the virus.
Conclusion
The COVID-19 outbreak is not the first pandemic and is unlikely to be the last. For the first time, however, our societies have the means to provide a coordinated, evidence-based, fair and global public-health response. While the efficiency of this response may partly depend on ML, it depends even more crucially on our ability to set up global collaborations and data-sharing agreements that can accelerate the discovery and validation of promising interventions.
The challenges of deploying artificial intelligence models in a rapidly evolving pandemic
The attention and resources of AI researchers have been captured by COVID-19. However, successful adoption of AI models in the fight against the pandemic is facing various challenges, including moving clinical needs as the epidemic progresses and the necessity to translate models to local healthcare situations.
The COVID-19 pandemic, caused by the severe acute respiratory syndrome coronavirus 2, emerged into a world that was seeing rapid developments in artificial intelligence (AI) based on big data, computational power and neural networks. In recent years, the gaze of AI researchers has increasingly turned to applications in healthcare. Inevitably, there has been much interest in exploring the potential for AI to support the response to the pandemic across a wide range of clinical and societal challenges1, for instance in disease forecasting, disease surveillance and antiviral drug discovery. However, to date AI has had surprisingly little impact on the management of COVID-19. This Comment focuses on examining the possible reasons behind the lack of successful adoption of AI models specifically for COVID-19 diagnosis and prognosis in frontline healthcare services. We highlight the moving clinical needs that models have had to address at different stages of the epidemic, and explain the importance of translating models to reflect local healthcare environments. We argue that both basic and applied research are essential to accelerate the potential of AI models, and this is particularly so during a rapidly evolving pandemic. This perspective on the response to COVID-19 may provide a glimpse into how the global scientific community should react to combat future disease outbreaks more effectively.
The evolving clinical need
The clinical management of COVID-19 has spanned various stages including anticipation, early detection, containment and mitigation, together aiming towards eventual eradication2. Each stage differs in its measured and actual disease prevalence, which directly impacts the availability of clinical resources, and over a matter of weeks, clinical priorities can fluctuate rapidly. Priorities may range from providing robust diagnoses, to maintaining infection control and ensuring availability of facilities for mechanical ventilation. These rapid changes, occurring in tandem with enhanced knowledge of virus behaviour and increasing availability of supporting data, have meant that the outputs required of predictive AI models need to constantly evolve. Accordingly, the AI models that are most urgently needed and can feasibly be built are likely to be different at each epidemic stage.
The anticipation and early detection stages
With a relatively low number of positive cases and many potentially asymptomatic cases during the early stages of the pandemic, a highly sensitive diagnostic AI model to detect COVID-19 would have been useful. The lack of pre-existing data from this new disease means the feasibility of building such new AI models to determine diagnosis or prognosis is a challenge, one that could be addressed by an AI community focused on breaking the existing barriers between data domains using machine learning. Generalizing AI models to unseen data (inference), data coming from different distributions (domain adaptation, transfer learning) and data with limited or no labels (semi- or unsupervised learning)3 are all priority areas in the technical development of AI. The early stages of a new disease have been described as overlooked periods in the general management of infectious diseases4. The AI community should design strategies and methodologies for rapid deployment in the event of future epidemic threats, to make data collection, model training, testing and wide deployment as efficient as possible next time.
The containment and mitigation stages
During the containment and mitigation of COVID-19, data have become increasingly available with the exponential growth of confirmed positive cases. During this time it is essential to rapidly curate sizable training datasets and develop stable, well-performing AI models that can respond to emerging clinically urgent needs, such as rapid, consistent patient triage at scale across a health service.
Reverse transcription polymerase chain reaction (RT-PCR) tests via nasopharyngeal swabbing have nearly 100% specificity and are considered the diagnostic ground-truth for COVID-19. However, RT-PCR has limited negative predictive value with variable availability and diagnostic speed. Alternative methodologies for diagnosing COVID-19 include medical imaging techniques such as computed tomography (CT) and chest radiographs5. Some groups have also explored point-of-care ultrasound, albeit with limitations6. Driven by data availability, the focus of AI work in COVID-19 has centred on RT-PCR-labelled diagnostic models7, or the automated evaluation of clinical/imaging features — for example, lung involvement on CT imaging8. Those developing AI-assisted diagnostic tools must recognize that very high diagnostic accuracies are required to demonstrate added value above and beyond existing clinical imaging and RT-PCR tests.
It is also important to question which prognostic outcomes require greatest prioritization during this period. The majority of existing AI models aim to predict hospitalization and mortality9 using predictors such as age, gender, blood biomarkers, pre-existing co-morbidities and imaging5. In resource-constrained clinical environments, there is great value in predicting resource consumption as a ‘surrogate’ prognostic outcome, as a lack of personal protective equipment, for example, can directly affect community prognoses10. Intuitive candidate outcome measures for AI models might include time spent on mechanical ventilators or within intensive care units. But as knowledge of COVID-19 has grown, early intubation of a patient has diminished in priority in the care pathway. Similarly, with limitations in resources, pragmatic choices have had to be made regarding patient selection for intensive care unit admission. Evolving management strategies such as these have a real-time impact on the outcomes that AI models aim to predict. Disease progression (or regression following treatment) models can be trained using time series data, such as longitudinal CT images11, to quantify the likelihood of developing severe pneumonia and acute myocardial injury, two leading causes of mortality12, and the cytokine release syndrome. A lesson from the COVID-19 pandemic has been that AI models motivated merely by the practical convenience of acquiring available labelled data has had limited clinical value.
The eradication stage and beyond
At the later eradication stage, constraints in data availability, development time and clinical resources would gradually be eased. The number of positive cases can drop quickly. Yet the need for a real-time, convenient, highly sensitive screening tool may persist to control transmission and judiciously recognize potential outbreaks.
The requirements for prognostic tools may shift to the identification of patients at risk of developing long-term health problems such as pulmonary fibrosis. Indeed cardiopulmonary, neurological13 and urological14 damage are all being recognized following COVID-19 infection. Given the potentially significant health service resource requirements that may result from long-term complications across large swathes of the population, the post-acute phase of COVID-19 will be a critical clinical research area, where AI models may play a central role.
Translating AI models
A typical AI translation workflow (Fig. 1) includes model development, model deployment and model adaptation (or model update). The COVID-19 AI research efforts have been concentrated primarily on new model development and the urgency brought about by the pandemic must not override the stringent requirements for clinical deployment15. Despite time pressures, rigorous validation is key to ensuring that safety and efficacy are tested; models must be validated before initial deployment and continuously monitored and adapted when implemented in local healthcare environments and as outcome likelihoods change due to evolving patient management strategies. Failing to adhere to such practice will impede translation and compromise the impact of AI on clinical needs.
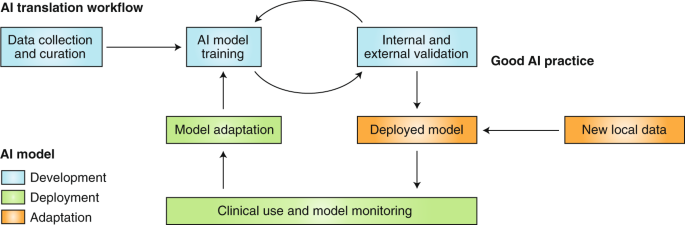
Pre-deployment validation
Recent COVID-19 AI models have been criticized for a lack of transparency in development and a high likelihood of bias towards non-representative patient populations5. Limitations in data availability and quality can be the inherent cause of problems — for example, validation datasets with unrealistically high numbers of control cases acquired at the start of an outbreak or extremely low numbers of control cases at the peak of the outbreak. These models are unlikely to be directly useful in all stages of the pandemic due to potential bias.
Best practices in rigorous design and analysis of experiments should be adopted for AI model validation. In addition, model interpretation methods help to explain the reasoning of the predictions16,17, and may also indicate when certain data-driven methods are unlikely to generalize18. Model transparency could also be key to addressing regulatory and ethical issues19,20.
Local adaptation
It is not uncommon to find that an AI model trained with data from one healthcare centre, or even from multiple centres, does not generalize as well at a new centre. For example, the accuracy of chest X-ray detection, represented by the area under the receiver operating characteristic curve, was significantly reduced from 0.93 in a multi-centre internal validation to 0.82 on external validation data18. The practice of pre-deployment external validation reduces the risk of this overfitting problem based on the assumption that external data represent new local data. However, for each individual healthcare environment, local data are likely to have unique characteristics due to centre-specific acquisition features, equipment and protocols, all of which may have differing clinical constraints and requirements21. Moreover, temporal differences in data may increase, adversely affecting model accuracy, as the demographic and immunity landscape and clinical practice shift between different stages of the pandemic22. AI models therefore should have a continuous monitoring and adaptation strategy to these changing data to maintain their predictive accuracy.
Most proposed AI approaches for COVID-19 diagnosis/prognosis have so far been ‘locked’ algorithms that do not facilitate future adaptation. Model-adapting methods from other medical applications should be tested and integrated in these developments, such as transfer learning23 and model retraining with a small local dataset. Recently, the US Food and Drug Administration has proposed a new approach to allow AI-based software to adapt and improve from real-world use24, paving the regulatory pathway to address these local adaptation needs.
Conclusion
The COVID-19 pandemic has presented numerous challenges to virtually every section of society in all geographic locations. AI can be an enabling technology to support urgent clinical needs in disease diagnosis and prognosis but is reliant on appropriate infrastructure, data management and translational pathways. New international cross-disciplinary collaborations, carefully identifying time-, course- and region-dependent clinical actions in response to COVID-19 can benefit from scientifically sound AI model development, validation and deployment to support local healthcare providers. Safe and responsible translation is the only way to realize the promise of AI models to contribute to combating the current coronavirus pandemic, its aftermath and potential future clustered outbreaks or comparable healthcare emergencies.
Nanoscale nights of COVID-19
As the spread of SARS-CoV-2 has triggered worldwide closures of research labs and facilities, Kostas Kostarelos shares his views on what may be going wrong in the fight against COVID-19 and how the nanoscience community could and should contribute.
The beauty of viral nanoparticles
During several years of teaching nanomedicine, I have illustrated the uniqueness of viral nanoparticles to many generations of students: “Remember, viruses are the most beautiful, smart and capable nanoparticles!” I have always enthusiastically highlighted their structurally beautiful nanoscale features, and their biological trickery in transferring their genetic contents into target cells and hijacking them to express proteins. Viruses are evolutionary works of art and in the fields of gene therapy, vaccinology and immunotherapy (to name just a few), scientists are engineering and using viruses for the smart delivery of molecules and genetic information. However, I must admit that during the past few late nights, while working in my makeshift home office, I cannot help but feel strangely guilty for my past opinions and lecture notes. As I gradually get more gloomy looking at the daily updated counts of ‘confirmed cases’, ‘deaths’ and ‘recovered cases’, guilt starts taking over. How can such beautiful nanoparticles create so much human loss, havoc and devastation? How can such nanoscale beauty so rapidly metamorphose into a wild beast?
The human factor
We knew this was coming. Experts and international committees have long been analysing and developing scenarios and plans to be able to respond to a potential intentional or unintended virus outbreak. There were also warnings that owing to our excessively industrialized agricultural and animal farming activities, climate change, highly congested urban areas and international aviation, which has created a ‘smaller’ and more accessible world, the risk for the spread of a contagious virus or an antibiotic-resistant bacterial strain was almost certain to happen. Not only were we told, but we had been given warning signs throughout the past 20 years, including avian influenza virus (Hong Kong, 1997), severe acute respiratory syndrome (SARS) coronavirus (China, 2002), swine influenza virus (Mexico, 2009) and Middle East respiratory syndrome (MERS) coronavirus (Saudi Arabia, 2012). These magnificent nanostructures that combine structural complexity with biological efficiency (Fig. 1), have all been originally transmitted from different animal species to humans; importantly, they are highly contagious with human-to-human transmission, which has sadly caused many deaths. Unfortunately, we collectively failed to understand that the risk was clear, imminent and significant. We failed to guarantee that the plans and directives, developed by experts, can be rapidly and effectively implemented in case of an outbreak. Indeed, each efficient viral infection is met by a multiplicity of human inefficiencies. We were out-beaten by nature’s nanostructures.
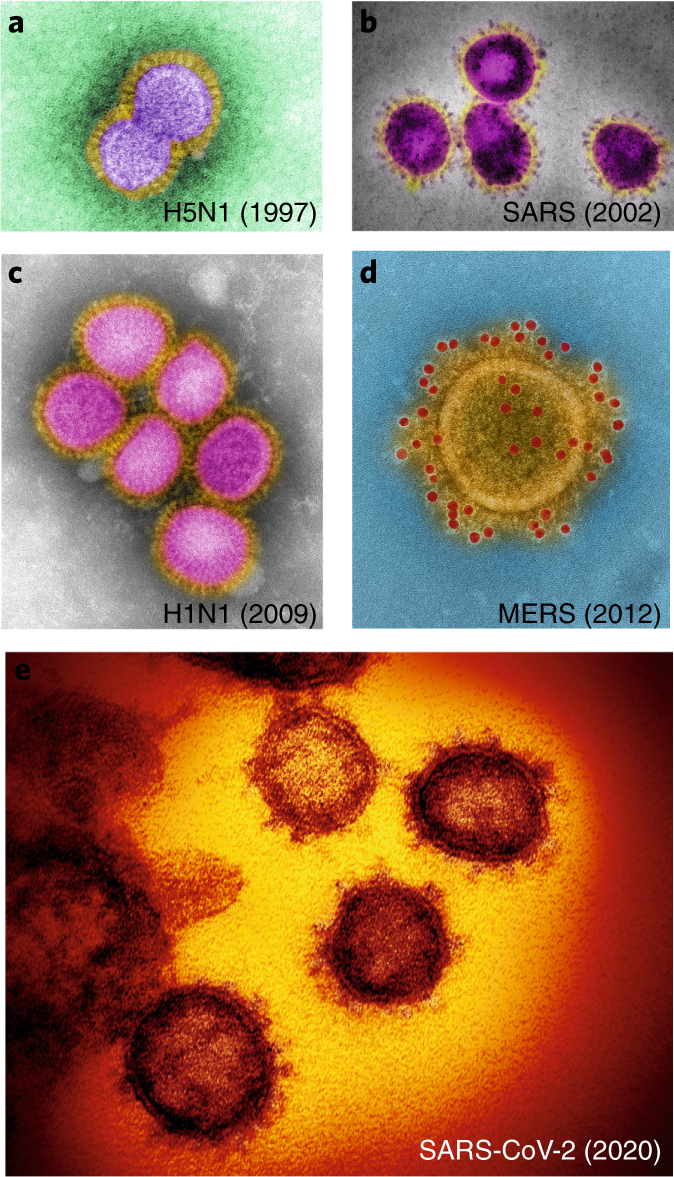
The scientific facts
The acute need is to get the scientific facts right, as fast and accurately as possible. We need to take advantage of the technology and automation in our laboratories as well as powerful modelling approaches to reveal scientific facts about COVID-19. What have we already learnt? SARS-CoV-2 is very similar to previously studied coronaviruses. It binds to angiotensin I converting enzyme 2 (ACE2) cell receptors and is highly transmittable among humans, even at the latent stages of infection. We know that SARS-CoV-2 infects the upper respiratory system and, in particular, the lung epithelium, with a severe downstream effect or direct impact on the myocardium. Infections of older (male more than female) individuals, and of patients with chronic underlying (diagnosed or not) conditions are the most vulnerable. Lastly, we know that 80% of infected individuals are asymptomatic or suffer from mild symptoms, 15% need hospitalization and 5% develop a serious illness and may need critical care. We also know though, that valid epidemiological data should be taken with great caution as different countries and health systems have very different approaches to testing and reporting.
However, there is also a lot we do not know. We do not know whether SARS-CoV-2 is able to mutate into different serotypes and how rapidly. We do not know whether cell internalization and viral transduction of SARS-CoV-2 is dependent on temperature, multiple rounds of infections and/or generations of virus progeny. We do not know whether the antibodies and titres produced in infected individuals are able to confer effective immunity against subsequent exposures to SARS-CoV-2 and for how long such immunity may last. Finally, we need to agree on strict parameters for the clinical reporting of COVID-19 infections and outcomes, which all national health agencies should adhere to. This is the only way to determine valid transmission, fatality and protective immunity rates. Only if we generate accurate scientific knowledge (even if inconclusive), can we assess the validity of public health interventions that are currently in place in many countries around the world, and potentially reduce the demand for such dramatic societal sacrifices.
Where have all the (nano)scientists gone?
In order to generate the urgently needed scientific knowledge, we need to get our technically capable, bright, young scientists back in the labs. I cannot help but wonder, why most governments in Europe (and more recently also in the USA) ordered the closures of world-class research laboratories and their support systems, during a generational crisis of biological and biomedical emergency. Many trained biological or biomedical scientists have been sent home, their laboratories closed down and their reagents left to expire. Of course, scientists would have to avoid working in confined spaces, should wear protective gear and would have to be regularly monitored for infection — but we need our laboratories back. Our energies and efforts should be focused on how to contribute to the fight against COVID-19. We — the biomedical scientific community — have the responsibility to support our clinical and healthcare colleagues, who work on the frontline of the pandemic, with our technical and intellectual capabilities in generating relevant and much needed scientific knowledge. We need to maximize synergies free from any political, linguistic, financial, geographical or scientific discipline obstacles. It is shameful that highly trained scientists are considered ‘non-essential’, while our nurses and clinical colleagues are daily exposed to the virus in their efforts to treat a constantly increasing number of patients.
The cancer analogy
Many heated conversations are currently taking place as to the best way to manage the COVID-19 menace. As I have tried to follow the different opinions, I cannot stop thinking of an analogy to cancer nanotechnology, that is, the three key principles in managing an individual cancer patient: early detection, monitoring and targeting. These principles, if exercised simultaneously, could also be illuminating as a way forward in the management of the COVID-19 pandemic. Early detection has improved the prognosis of many cancer patients. Similarly, early detection of individuals and groups, who are infected with COVID-19, could substantially accelerate the ability to manage and treat patients, but also infection hotspots. All chronic conditions, such as cancer, are further managed by regular monitoring. Therefore, monitoring should be undertaken not only for patients already infected with COVID-19, to track progression and responses, but also for healthy essential workers to ensure that they remain healthy and to reduce the risk of further spreading. Finally, nanomaterials (as well as other biologicals, such as monoclonal antibodies) are often used for targeting therapeutic agents specifically to cancer cells to minimize damage of healthy tissue. The same principle of targeting should be applied in the management of COVID-19 to be able to effectively isolate and treat infected patients. But also to establish a ‘protective targeting’ strategy to shield the vulnerable segment of the population (by isolating them or by social distancing, but with provision of emotional and practical support), and protect ‘essential’ workers (by making sure that protective gear and monitoring is provided). Only if all three principles are applied, the rest of society can and should return to normal function to support the activities in managing the pandemic. In this way, I want to finish by paraphrasing an undergraduate cancer management textbook: The COVID-19 pandemic will become a chronic disease, and as for any other chronic medical condition, COVID-19 stricken societies have families, jobs, businesses and other commitments. Therefore, our aim is to cure COVID-19 if possible; however, if not curable, we need to control the symptoms to improve the quality of patients’ lives by assuring society’s function to be able to support its ill and vulnerable.
Artificial intelligence cooperation to support the global response to COVID-19
In an unprecedented effort of scientific collaboration, researchers across fields are racing to support the response to COVID-19. Making a global impact with AI tools will require scalable approaches for data, model and code sharing; adapting applications to local contexts; and cooperation across borders.
As of 24 April 2020, the SARS-CoV-2 virus had infected 823,626 people with more than 40,598 confirmed deaths, and with nearly every citizen of the planet at risk1. Given the need to interrupt disease transmission and the increasing scale of the pandemic, digital technologies are relevant to support the fight against the virus. Among these technologies, machine learning and artificial intelligence (AI) methods are being applied in many COVID-related domains, resulting in approximately 200 preprints and scientific articles from 1 January 2020 to 24 April 20202. However, for AI applications addressing COVID-19 to have a global impact, large-scale data and model sharing, operational validation, and adaptation to local contexts are needed. This requires cooperation and solidarity across borders as well as the involvement of many relevant parties, including healthcare workers.
Taxonomy and applications
In a review of the emerging literature at the intersection of AI and COVID-19 by some of us3, a framework to categorize multidisciplinary research on three scales is proposed: molecular, clinical and societal (epidemiology and infodemics).
Molecular applications include protein structure prediction4, improving viral nucleic acid testing5, drug repurposing6 and drug discovery7. These applications draw on a range of AI methods including the construction and mining of biomedical knowledge graphs, deep learning architectures designed to predict characteristics of protein structures or protein–ligand binding affinities, text modelling approaches applied to genetic sequences, and the use of reinforcement learning for drug design.
Clinical applications to improve patient care range from diagnosis to patient follow-up, treatment and outcome prediction. Deep learning techniques can help image-based diagnosis of COVID-19 from patterns in X-ray images and computed tomography (CT) scans8. Operational human-in-the-loop AI systems have been developed to help radiologists reduce the time needed to review scans. Smart watches, mobile phones and other wearables can potentially be used for disease tracking9, along with other remote monitoring applications that allow doctors to follow patients remotely, saving time and personal protective equipment. Combining different data sources like health records and medical imagery can help predict patient outcomes10 and could be used to project the needs of hospitals and healthcare systems such as surges in demand for intensive care unit beds. The use of robotics in telemedicine and in other tasks like cleaning and sterilizing medical facilities could also prove valuable.
For epidemiology, AI can improve early warning systems and the modelling of empirical data11. In particular, AI can be applied to understand and simulate public policy interventions such as quarantining, social distancing and de-escalation strategies, and augment classical epidemiological techniques by approximating functions for which analytic transmission equations are not known12,13. Additionally, unsupervised clustering techniques and risk-scoring algorithms, which are able to combine disparate data sources, may help identify similarities between regions, and forecast which areas will require more resources.
For infodemics, AI can be used to fight misinformation and disinformation by managing the current overabundance of information, which creates confusion and makes it difficult to identify trusted sources14,15. AI methods can support audience analysis (for example, social media, TV, radio) and accelerate fact checking16. Social media analysis, for example, is providing insights into global trends and sentiment around the pandemic and its socioeconomic impacts17. Research is being conducted to identify the emergence and escalation of hate speech and verbal attacks against minorities and communities suffering from discrimination that may result in violent action or their exclusion from access to healthcare18. Virtual assistants and chatbots are being used to disseminate trusted content at scale19, requiring mechanisms for propagation of updates to information as new evidence becomes available in the COVID-19 response.
Data and model sharing
AI applications require data. There are currently dozens of data-sharing initiatives around COVID-19 at the three application scales, spanning the international, national and local levels. These datasets include: genetic sequences20, genome analysis21, protein structures, patient clinical data, medical imagery, case statistics, epidemiological data22, mobility data, social media posts, news articles and scientific literature23. The hyper-fragmentation of data-sharing efforts is a challenge, as it might result in advances that are restricted to particular projects and local communities. Establishing scalable approaches for data, model and code sharing could accelerate the development and dissemination of new applications. At this stage, global, open, comprehensive, comparable and verified data-sharing initiatives will be beneficial to connect and promote cooperation between disparate communities and geographies24.
Open science can accelerate knowledge dissemination and capacity building of national health systems, supported by multi-stakeholder AI partnerships operating across international borders. For example, the data platform of the Epidemic Intelligence from Open Sources (EIOS)25 network enables early detection, verification and assessment of public health risks and threats using open source information. Under the philosophy of collaboration and not competition in early detection, the community of practice for public health intelligence includes governments, international organizations and research institutes that collaboratively assess and share information about outbreak events in real time. The EIOS network picked up the first article reporting an unknown pneumonia cluster in Wuhan at 3:14 UTC on 31 December 2019. From the epidemiological perspective, global standards and interoperability between databases could enable coordinated response and decision-making at global, national and local levels. Understanding the epidemiologic features and risk characteristics of different demographics as we move into different phases of the pandemic will require accounting for health system resource capacities, public health interventions, environmental factors and the social implications of COVID-19.
Beyond data sharing, few initiatives currently share trained AI models related to any of the proposed applications. Barriers to be overcome include constraints due to specific computational, architecture and infrastructure requirements; a lack of documentation; validation and explainability aspects; and legal concerns regarding privacy and intellectual property. Sharing pre-trained and validated AI models could accelerate the adaptation of solutions to local contexts. Examples of models that could be widely applicable include those used to diagnose illness from imagery, predict patient outcomes, filter misinformation and disinformation based on spreading patterns through social networks, or distil knowledge graphs from large collections of scientific articles. Getting to a future of open AI models will require AI governance frameworks that take into account ethical, social, clinical, legal and commercial considerations.
From research to impact
One of the challenges of making a greater impact with AI applications is not knowing where to start and how efforts can be most effective. There is a need for greater cooperation between practitioners and the AI community. The AI community already is, and should continue to seek advice and guidance from domain experts, including government officials, healthcare professionals and first responders, among others. They can help flag processes that are good candidates for automation. These include repetitive or time-consuming tasks that involve finding patterns in rich image, video, audio or biomedical data — for example, CT scans — or tasks that involve synthesizing massive datasets from sources such as symptom tracking systems or social media channels.
To date, few of the research projects and systems presented are as yet sufficiently mature to be operationalized at scale, with different applications having different timescales of development, validation and deployment. As a result, it is important for the founders, users and AI community to map which technologies could assist with the short-term response, mid-term recovery efforts, and the longer-term preparedness for future pandemics.
Developing solutions for the current pandemic requires coordination and prioritization26, focused on key unmet needs that account for operational realities. When possible, solutions should be based on adapting already validated systems27 and should avoid overburdening an already stretched healthcare workforce with new tools that may not always help to improve outcomes. Furthermore, it is important that new and existing solutions are adapted to fill the specific needs and contexts of low- and middle-income countries. Applications dealing with clinical and patient data should not ignore the existing regulatory frameworks designed to ensure quality, security and safety, and minimize potential risks and harms. Despite the urgency of the fight against COVID-19, rigorous assessment of potential solutions is required, which in some opportunities can be fast-tracked without increasing risks.
Collaborations among global health actors, private sector partners and other stakeholders are already forming to accelerate the development, production and equitable global access to new health technologies essential to COVID-1928. Coordination and cooperation between regulatory bodies and international and regional organizations would aid in channelling research on the ground, and would assist in implementing systems in countries with weaker frameworks and governance structures. Compliance with reporting guidelines29,30 would enable more systematic and efficient reviews of evidence, and comparisons of healthcare-related AI applications along the dimensions of safety, clinical validity and performance accuracy.
The global fight against the COVID-19 infodemic should be treated as a scientific challenge on a par with the spread of the disease itself, since behavioural change is critical to the pandemic response. Media and information literacy remain critical, and there is a need to quantify individual rates of exposure to misinformation and disinformation. Infodemic management frameworks can be used to support timely translation of evidence into knowledge and advocacy adapted to local cultures, languages and contexts. AI methods and tools should be used to fill the health information gaps for individuals and policymakers alike for a whole-of-society response based on evidence and science. To effectively combat the infodemic while ensuring the fundamental right to access to information, the international community must share and amplify good practices, identify priorities, facilitate partnerships, and provide advice and technical assistance to governments and relevant national stakeholders to foster international cooperation. The pandemic is stoking xenophobia, hate and exclusion, posing a far-reaching — and potentially long-lasting — threat to human rights31. Understanding the dynamics and spread of hateful speech will help design more effective interventions and change the narrative.
Global challenges and digital cooperation
We now have the ability to create new AI tools to address age-old problems. But having the means to create such applications doesn’t necessarily mean we should. Any AI application that could be used in the fight against COVID-19 ought to undergo an assessment to ensure that it complies with ethical principles and, above all, respects human rights. Even when following privacy-by-design and ethics-by-design guidelines in the development and deployment of AI-based solutions, practitioners will be confronted with questions on how to protect fundamental rights, including the right to privacy. When rolling out any of the above proposed AI solutions at scale, we must ensure they do not breach global commitments to protect rights and freedoms, including commitments to non-discrimination, preventing mass surveillance, and protecting journalistic sources. In order to do so, stakeholders should ensure that principles, including those of openness and accessibility, are at the heart of AI-enabled solutions. AI applications in health should also promote fair and equitable global access, supporting member states’ commitments to achieve universal health coverage.
The global nature of the pandemic requires global solutions, but there is also a need for local adaptation to account for biases and contextual factors. For instance, a system to diagnose COVID-19 pneumonia in chest X-rays will need to be trained differently in countries with different levels of prevalence of diseases or conditions with related image patterns, such as tuberculosis and HIV. Similarly, epidemiological models tailored to pastoral areas, island states, refugee camps or conflict zones should take into account different socioeconomic, cultural and structural variables than the ones described in scientific literature — which have been developed mostly in China or in Western countries. Likewise, chatbots to inform citizens about public health measures require specific natural language processing models, and often vast amounts of training data, which today are available for just a few hundred of the more than 7,000 living languages. The participation of researchers and local data science collaboratives from underrepresented regions including Africa and Latin America, for example the Data Science Africa network, will be critical to ensure that any AI system has a positive impact at global scale. With this in mind, solutions may need to be designed with low-bandwidth requirements up front — for example, medical diagnosis apps without need for connectivity and that do not compromise privacy or data security. Such applications represent an opportunity to promote edge computing for AI and other energy-efficient approaches for the responsible use of computational resources.
The role of AI in providing meaningful solutions to the current pandemic has yet to be defined. However, there is a clear need for a globally inclusive research agenda to direct action against this pandemic — and future ones — without leaving anyone behind. It is reasonable to believe that the world will be more digital than ever in the post-coronavirus era, and that AI will increasingly become one of the driving forces shaping our society32. This pandemic underscores the urgency for stakeholders to effectively translate ethical principles for AI into practice33. ‘Solidarity’ is the name of the international clinical trial launched by the World Health Organization to help find an effective treatment for COVID-1934. It is the sense of solidarity with our neighbours that encourages physical distancing, which is vital to suppressing transmission of the virus within communities. Similarly, solidarity should be a core principle guiding the development of innovative and ethical AI applications to fight the COVID-19 pandemic, and help advance the global Sustainable Development Agenda
A nanoscale arsenal
Nanomaterial design and strategies are at the forefront in developing advanced antiviral and antibacterial therapies.
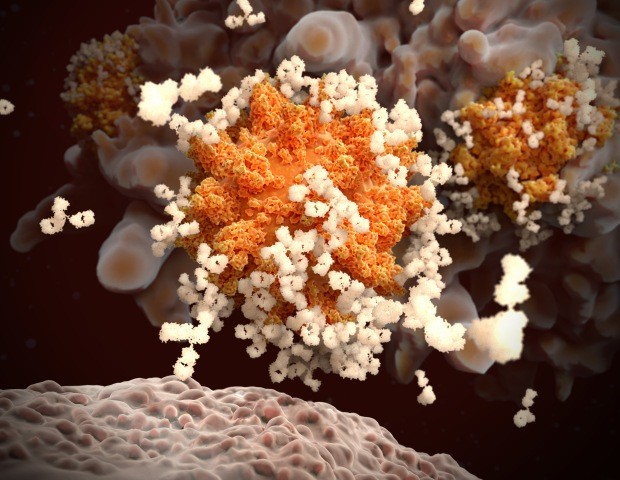
The world has made tremendous progress in science, technology and medicine since the last epidemic of Spanish flu a century ago; still, our ill-preparedness for a pandemic has forced us to look for better alternatives and strategies. We have already faced several viral outbreaks such as Zika virus, Ebola virus, and swine flu, as well as the long fight against tuberculosis (TB), HIV, and hepatitis C (HCV) in the last century. These experiences have taught us that although microbes and humans have co-existed and interacted with each other, we must be mindful of risk, and that no matter how aware and prepared we are, we must face the unexpected and develop broad-spectrum strategies to emerging microbial threats.
Can nanotechnology provide fast, accurate and cost-effective diagnostic tools?
The COVID-19 challenge comes from the fact that asymptomatic infected people may be a significant source of spread, as with TB, HCV, or even HIV, where early and accurate diagnosis at initial stages of the infection can lead to better management and treatment.
Let us understand this by comparing HIV and COVID-19. AIDS caused by HIV is one of the most significant challenges of this century. An estimated 690,000 people died from AIDS-related illnesses in 2019. In the absence of any groundbreaking treatment, early diagnosis remains key to prolonging life. In countries such as India, with a huge population, significant poverty, and sub-standard healthcare system, the challenges are more critical.
Even after 40 years of research, ELISA based diagnosis (enzyme-linked immunosorbent assay), which has several limitations, is still the gold standard. Biosensors based on nanotechnology are regarded as a great hope to overcome these challenges. Their benefit comes through their unique properties, such as small size (1—100 nm, matching these microbes), shape (can be designed to mimic the micro surface), ease of function (chemically tailorable), high sensitivity (high surface to volume ratio), and robust structure. Several nano-based sensors are already being developed and tested based on biochemical, electrochemical, electromagnetic conjugated with molecular technologies for HIV biomarker detection.
COVID-19 was effectively controlled in some countries, such as South Korea and Hong Kong, due to robust testing. Early in the outbreak, governments across the world used rapid COVID-19 antibody tests to diagnose infection, but many failed to detect the stage of infection. Later, more evolved tests using nanotechnology (colloidal gold method) that can detect very low viral loads with high sensitivity were developed, which helped in controlling the spread of infection and suitable treatment paths. Nanotechnology is poised to revolutionize the diagnosis of pathogens by enabling safer, faster, reliable, cost-effective and sensitive nanodevices and nanoprobes for microbial detection and screening.
The tools of prevention
Researchers have been working constantly to find a vaccine — and nanotechnology is among their strongest tools. To outline the process: viral or bacterial infections generate an immune response (antibodies) by recognizing virus/ bacteria proteins on the surface (antigens) that neutralize these pathogens. Blood therapyusing plasma of recovered patients (used in India for COVID-19 treatment) is based on this concept, where the transfused plasma contains high levels of virus-neutralizing antibodies.
Vaccine development involves the same logic where a viral/bacterial mimicking immunogenic material is administered to generate a strong immune response without causing disease symptoms. The immune response of a potent vaccine depends on antigen stability, delivery and presentation. Over the last decade, nanomaterial-based vaccines have provided compelling advantages in vaccine development by increasing antigen stability, multivalent presentation to B cells leading to strong and enhanced immune response. Several nanoparticles, including self-assembled protein nanoparticles, organic nanoparticles, inorganic nanoparticles, and liposomes have been explored to generate an immune response.
The earliest example of nanoparticle-based vaccines used ferritin for developing influenza, HIV, hepatitis B and hepatitis C virus vaccines. In the case of COVID-19, the development of a modular nanoparticle-based vaccine, where the antigen within is interchangable, showed recent signs of progress for rapid vaccine development. It also showed that the vaccine developed a 10-fold higher antibody titre when used at five times less dosage than a soluble antigen. The results were validated in mice, and there are plans for human clinical trials in early 2021. Looking ahead, biomimetic nanomaterials hold promise for fighting infectious diseases, and could lead to the development of next-generation antiviral and antibacterial vaccines and therapies.
Antiviral and antibacterial therapy
In heavily populated countries such as India, infectious diseases due to bacteria, viruses, and parasites pose a serious public health threat Nanomedicine, with its unique capabilities, offers a modular and broad-spectrum antiviral and antibacterial therapeutic concept. We need to channel efforts effectively to develop targeted therapies against a broad spectrum of pathogens, rather than a single target whether it is COVID-19, HIV or hepatitis C.
Targeting strategies through broad-spectrum microbial and host targeting will provide multilateral solutions to current, as well as emerging microbial threats. Regarding targets, although each virus or bacteria has a unique structure, they share some similarities, which need to be identified and targeted. Nanomaterials can carry multitargeted entities, thus making it easier to target a broad spectrum of microbes through direct microbe targeting or stopping host cells or both.
A few examples using this approach include nitric oxide-releasing nano antibiotics, that can target bacterial cells through multiple paths. Other examples include interferon-lambda (IFN-λ), nanomaterial-enhanced replication inhibitors, membrane inhibitors and so on. Also, the therapies can be designed and delivered in a precise manner to have higher efficiency, specificity and sensitivity. It is important to understand that to make these concepts work needs a coordinated effort of biologists, engineers, material scientists and the private sector industries
Pre-emptively inactivating pathogens
Though treatment through antiviral, antimicrobial therapies or vaccines is the first-line approach to treat these microbial infections, rendering these infectious agents inactive before entering the host will play a major role in combating future pandemics.
Contaminated surfaces in public spaces are a major conduit for the uncontrolled outbreak of these infectious diseases. Nanoformulation based coatings that can be antimicrobial, or release antimicrobial agents on demand, have provided a new perspective to fight the pandemic causing agents.
These systems can be smart and responsive, releasing antimicrobial agents upon stimuli such as touch, change in temperature, or rubbing, making them an efficient tool to fight the spread of infection. Nanomaterial based PPE kits, nanoengineered masks for healthcare workers and the general public will provide additional comfortable, resistant, and safer means of protection against these microbes.
Technical Institutes in India, including IIT Kanpur, have exploited nanotechnology to develop nano-based products such as masks and PPE kits, which have been praised. However, these scientific efforts require effective coordination and collaboration among various stakeholders from government agencies to private partners to be successful.
Lessons learned and the way ahead
Nanotechnology can be a great weapon to fight future pandemics on various fronts. We have to find innovative solutions using this tool to track, target and eliminate the threat of these deadly microbes. Other technologies will complement nanotechnology to develop an innovative engineering solution in this global fight against existing as well as emerging microbial threats. We see an optimistic future where a coordinated effort from scientists of varying backgrounds will help to control some of the biggest problems of medical science, including microbial infections.
AI vital in finding new targets for existing drugs
Artificial intelligence is harnessed to accelerate drug repurposing in the search for COVID-19 solutions.
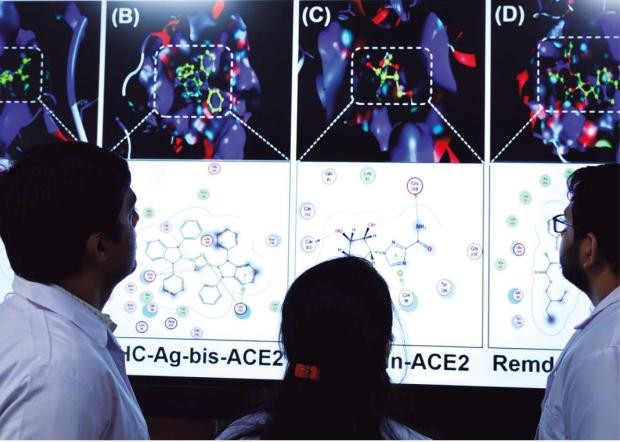
The use of artificial intelligence in the journey of new drug discovery has become integral to their development. Advances in computer modelling of biological processes, or ‘in-silico biology’ have revealed some of the crucial aspects of effective drug molecules with respect to their structure, functional groups, physiochemical nature, permeating ability, binding potential, bioavailability, receptor behaviour, and genetic interaction. In combating the spread of COVID-19, a broad range of strategies are being assisted with in silico validation, including small molecular drug repurposing, enzyme inhibition, and target drug delivery.
Among these, drug repurposing is a useful strategy to identify newer therapeutic applications. Repurposing hastens the drug development process, and has therefore received considerable research attention. Clinical investigations on repurposed small molecule drugs show promising response for COVID-19 therapy. Enzymes that play a role in the pathogenesis of SARS-CoV-2 virus include serine protease (TMPRSS2), cysteine protease (cathepsin B and L), RNA-dependent RNA polymerase (RdRp), 3-chymotrypsin-like protease (3CLpro), papain-like protease (PLpro), among others.
For most of the current US Food and Drug Administration (FDA) approved molecules under therapeutic regimen for COVID-19, the enzyme 3-chymotrypsin-like protease (3CLpro) has been identified as a promising drug target. Drugs including remdesivir, ertapenem, imipenem, and tenofovir reveal significant inhibitory interactions with high binding affinity on 3CLpro active sites. Therefore, these drugs, either alone or in combination, are under investigation for the management of COVID-19. Clinicians working to fight the COVID-19 pandemic are exploring repurposing existing drugs via in silico screening of viable candidates.
3CLpro renders high biological significance for the virus to synthesize its polyproteins emerging from open reading frames, the part of nucleic acid available for transcription. In addition, 3CLpro shares with the majority of human coronaviruses a sequential homology with respect to its structural and functional groups. It should be noted that 3CLpro is involved in cleaving 11 prominent sites to yield 16 non-structural proteins (NSP). Hence, targeting 3CLpro with novel enzyme inhibitors appears to be an ideal choice for researchers to minimize the viral replication time. Residual amino acids (His 41, Phe 140, Gly 143, Cys 144, Cys 145, His 163, Glu 166, His 172) spread across the catalytic domain (I & II) of the enzyme seeks the attention of the cleavage process. The challenge therefore is to engage all the active sites involved in mediating the production of NSPs to alter the sequence of replication.
Recently, remdesivir, an investigational small molecule entered the frame for therapeutic management of COVID-19. Immunologically, remdisivir has proven anti-viral efficacy against a spectrum of RNA viruses that includes SARS-CoV, MERS-CoV, Ebola virus, Nipah virus and other strains of bat coronaviruses that are capable of infecting human respiratory epithelial cells. Hence, it was believed that treatment with remdisivir offered promising therapeutic benefits to the patients with COVID-19.
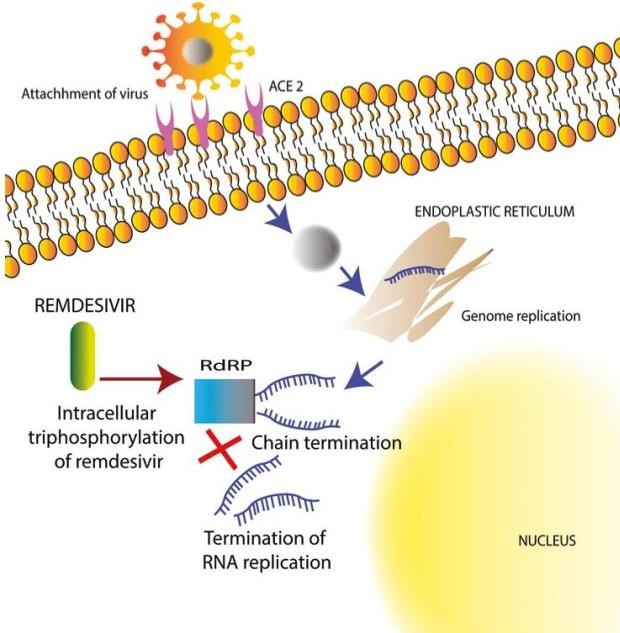
Remdisivir gained attention due to its diversified mechanism of action. Research suggests that remdisivir acts as nucleoside analogue and inhibits the enzyme RdRp (RNA-dependent RNA polymerase), limiting the replication of SARS-CoV-2 virus in the host cell. Although remdisivir was expected to be beneficial for a majority of patients, it still requires crucial validation on whether its pharmacology against multiple drug targets can be extended.
Apart from RdRp and 3CLpro, another enzyme, trans membrane serine protease 2 (TMPRSS2), holds interest for researchers as it primes the receptor binding domain of the viral S protein and is involved in cleavage of S1-S2 bonds to set the virus free from angiotensin-converting enzyme 2 (ACE2) after gaining entry inside the cell. Such proteases significantly help in viral membrane fusion and release of antigenic RNA into the host cell cytoplasm.
Studies further reveal that the oral pancreatitis drug camostat mesilate is active by effectively blocking the production of inflammatory mediators like monocyte chemoattractant protein-1 (MCP-1) and tumor necrosis factor-α (TNF-α). Hence, combination therapy may render some more convincing recovery rates in COVID-19 patients than monotherapy. Conjugating camostat mesilate with remdesivir is a novel strategy that may inhibit the bioactive enzymes such as serine protease, RdRp and 3CLpro which in turn restrict the viral entry and suppress the replication mechanisms.
Drug repurposing is a necessary approach to discover new solutions.