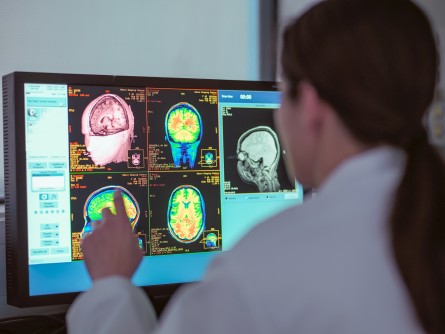
Doctors rely on imaging technologies that let them see inside the human body to diagnose diseases ranging from pneumonia to cancer. But access to these technologies is rife with inequities: low-income countries average just 1.9 radiologists per million people (the US has about one radiologist per 10,000 people). And within the US, patients in rural areas often have delays in imaging — and as a result, delays in disease diagnosis — because of a lack of equipment and physician shortages.
Fellow Sarah Hooper from the Fannie and John Hertz Foundation is helping develop a solution to both reduce diagnostic imaging costs and address shortages and delays in radiology: integrate automated, machine learning algorithms into the medical imaging workflow. Machine learning algorithms have the potential to transform medical imaging, from changing the way we build imaging systems to altering how images are analyzed and what information we can extract from them. During graduate school at Stanford, Hooper developed ways to improve the automated processing of cardiac MRIs. Now, as a research scientist at the National Heart, Lung, and Blood Institute’s (NHLBI) Imaging AI program, she’s working to integrate machine learning across a variety of imaging platforms.
“You can insert machine learning into any point of the imaging pipeline to make things more efficient,” says Hooper. “One program might make it easier to acquire images so you don’t need as much expertise to operate a machine; another might automate parts of the analysis workflow so that a radiologist can spend five minutes looking at an image instead of twenty. That all translates into patients getting results faster.”
As an undergraduate, Hooper worked with Drs Rebecca Richards-Kortum and Maria Oden at Rice University’s Institute for Global Health Technologies, studying how to reduce the cost of healthcare technologies for use in resource-limited settings. At the same time, during the classes required by her electrical engineering degree, she learned about the burgeoning field of machine learning — the branch of artificial intelligence (AI) that tries to teach computers to learn from experience the way humans do.
“At the time, there wasn’t much overlap in the resource-limited healthcare technology world and the machine learning world,” Hooper recalls. “But it was clear that these two worlds were going to coincide and that there was a lot of potential at their intersection.”
Hooper saw particular promise with automating medical imaging — radiology costs are high, the acquisition and analysis of scans is technically advanced, and imaging is critical to patient care. Hooper pursued her graduate degree as a Hertz Fellow in electrical engineering at Stanford University with the goal of working at this intersection and found mentors in both radiology and computer science.
“I had a very interdisciplinary mentorship team, and that was really enabled by my Hertz Fellowship,” Hooper says. “It enabled me to approach people from diverse fields and say ‘I’m looking for your expertise and I already have funding.’”
Over the next five years, Hooper developed algorithms that could learn to identify and label parts of medical images more easily than previous technologies. Specifically, she focused on cardiac MRIs —high-resolution images of the heart that doctors use to assess cardiac health. In standard image analysis pipelines, cardiologists often draw lines around different parts of the heart in many successive images to calculate key metrics about cardiac health, such as how much blood the heart is pumping over time. This “segmentation” of images into different parts by hand is time-consuming and can be inaccurate.
Hooper wanted a computer algorithm to carry out the segmentation. But machine learning algorithms must be trained: a computer is given data that humans have already analyzed to find patterns and learn how to automate the analysis. Previous machine learning algorithms that might have worked for cardiac MRI segmentation required a huge amount of training data from cardiologists before they could work on their own.
“The key technical challenge was figuring out how to do this without requiring weeks or months of clinicians’ time,” Hooper says.
Hooper successfully wrote new algorithms that could learn how to segment cardiac MRIs using far fewer already-analyzed images: about 100 instead of the 16,000 or more required previously. Researchers at the NHLBI, with whom Hooper collaborated, integrated these algorithms into a larger machine learning platform that is now being used by clinicians to analyze cardiac MRIs more easily.
Today, Hooper is herself a research scientist at the NHLBI’s Imaging AI program. She’s working more broadly across different imaging technologies, building an image analysis platform to help researchers and clinicians who want to segment many different kinds of images — from scans of the human body to pictures of cells growing in a dish or mouse organs being used to study disease.
“There might be pathology slides and we want to identify how many cancer cells there are on the slide, or we might want to calculate the size of a liver based on a CT scan,” Hooper explains. “For all these different images, the technical challenge of using machine learning to identify parts of an image is largely the same.”
Integrated with the image analysis platform, Hooper and the Imaging AI team are implementing additional machine learning algorithms to speed up image acquisition and improve image reconstruction. Ultimately, this platform will support machine learning algorithms throughout the imaging pipeline.