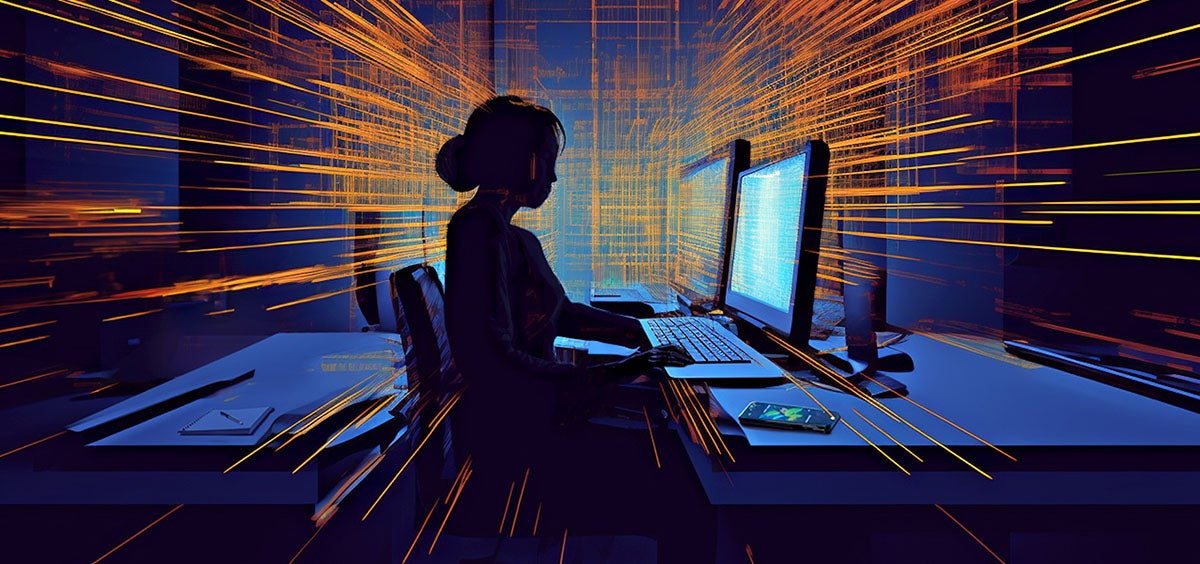
I still love software as much today as I did when Paul Allen and I started Microsoft. But—even though it has improved a lot in the decades since then—in many ways, software is still pretty dumb.
To do any task on a computer, you have to tell your device which app to use. You can use Microsoft Word and Google Docs to draft a business proposal, but they can’t help you send an email, share a selfie, analyze data, schedule a party, or buy movie tickets. And even the best sites have an incomplete understanding of your work, personal life, interests, and relationships and a limited ability to use this information to do things for you. That’s the kind of thing that is only possible today with another human being, like a close friend or personal assistant.
In the next five years, this will change completely. You won’t have to use different apps for different tasks. You’ll simply tell your device, in everyday language, what you want to do. And depending on how much information you choose to share with it, the software will be able to respond personally because it will have a rich understanding of your life. In the near future, anyone who’s online will be able to have a personal assistant powered by artificial intelligence that’s far beyond today’s technology.
This type of software—something that responds to natural language and can accomplish many different tasks based on its knowledge of the user—is called an agent. I’ve been thinking about agents for nearly 30 years and wrote about them in my 1995 book The Road Ahead, but they’ve only recently become practical because of advances in AI.
Agents are not only going to change how everyone interacts with computers. They’re also going to upend the software industry, bringing about the biggest revolution in computing since we went from typing commands to tapping on icons.
A personal assistant for everyone
Some critics have pointed out that software companies have offered this kind of thing before, and users didn’t exactly embrace them. (People still joke about Clippy, the digital assistant that we included in Microsoft Office and later dropped.) Why will people use agents?
The answer is that they’ll be dramatically better. You’ll be able to have nuanced conversations with them. They will be much more personalized, and they won’t be limited to relatively simple tasks like writing a letter. Clippy has as much in common with agents as a rotary phone has with a mobile device.
An agent will be able to help you with all your activities if you want it to. With permission to follow your online interactions and real-world locations, it will develop a powerful understanding of the people, places, and activities you engage in. It will get your personal and work relationships, hobbies, preferences, and schedule. You’ll choose how and when it steps in to help with something or ask you to make a decision.
“Clippy was a bot, not an agent.”
To see the dramatic change that agents will bring, let’s compare them to the AI tools available today. Most of these are bots. They’re limited to one app and generally only step in when you write a particular word or ask for help. Because they don’t remember how you use them from one time to the next, they don’t get better or learn any of your preferences. Clippy was a bot, not an agent.
Agents are smarter. They’re proactive—capable of making suggestions before you ask for them. They accomplish tasks across applications. They improve over time because they remember your activities and recognize intent and patterns in your behavior. Based on this information, they offer to provide what they think you need, although you will always make the final decisions.
Imagine that you want to plan a trip. A travel bot will identify hotels that fit your budget. An agent will know what time of year you’ll be traveling and, based on its knowledge about whether you always try a new destination or like to return to the same place repeatedly, it will be able to suggest locations. When asked, it will recommend things to do based on your interests and propensity for adventure, and it will book reservations at the types of restaurants you would enjoy. If you want this kind of deeply personalized planning today, you need to pay a travel agent and spend time telling them what you want.
The most exciting impact of AI agents is the way they will democratize services that today are too expensive for most people. They’ll have an especially big influence in four areas: health care, education, productivity, and entertainment and shopping.
Health care
Today, AI’s main role in healthcare is to help with administrative tasks. Abridge, Nuance DAX, and Nabla Copilot, for example, can capture audio during an appointment and then write up notes for the doctor to review.
The real shift will come when agents can help patients do basic triage, get advice about how to deal with health problems, and decide whether they need to seek treatment. These agents will also help healthcare workers make decisions and be more productive. (Already, apps like Glass Health can analyze a patient summary and suggest diagnoses for the doctor to consider.) Helping patients and healthcare workers will be especially beneficial for people in poor countries, where many never get to see a doctor at all.
These clinician-agents will be slower than others to roll out because getting things right is a matter of life and death. People will need to see evidence that health agents are beneficial overall, even though they won’t be perfect and will make mistakes. Of course, humans make mistakes too, and having no access to medical care is also a problem.
“Half of all U.S. military veterans who need mental health care don’t get it.”
Mental health care is another example of a service that agents will make available to virtually everyone. Today, weekly therapy sessions seem like a luxury. But there is a lot of unmet need, and many people who could benefit from therapy don’t have access to it. For example, RAND found that half of all U.S. military veterans who need mental health care don’t get it.
AI agents that are well trained in mental health will make therapy much more affordable and easier to get. Wysa and Youper are two of the early chatbots here. But agents will go much deeper. If you choose to share enough information with a mental health agent, it will understand your life history and your relationships. It’ll be available when you need it, and it will never get impatient. It could even, with your permission, monitor your physical responses to therapy through your smart watch—like if your heart starts to race when you’re talking about a problem with your boss—and suggest when you should see a human therapist.
Education
For decades, I’ve been excited about all the ways that software would make teachers’ jobs easier and help students learn. It won’t replace teachers, but it will supplement their work—personalizing the work for students and liberating teachers from paperwork and other tasks so they can spend more time on the most important parts of the job. These changes are finally starting to happen in a dramatic way.
The current state of the art is Khanmigo, a text-based bot created by Khan Academy. It can tutor students in math, science, and the humanities—for example, it can explain the quadratic formula and create math problems to practice on. It can also help teachers do things like write lesson plans. I’ve been a fan and supporter of Sal Khan’s work for a long time and recently had him on my podcast to talk about education and AI.
But text-based bots are just the first wave—agents will open up many more learning opportunities.
For example, few families can pay for a tutor who works one-on-one with a student to supplement their classroom work. If agents can capture what makes a tutor effective, they’ll unlock this supplemental instruction for everyone who wants it. If a tutoring agent knows that a kid likes Minecraft and Taylor Swift, it will use Minecraft to teach them about calculating the volume and area of shapes, and Taylor’s lyrics to teach them about storytelling and rhyme schemes. The experience will be far richer—with graphics and sound, for example—and more personalized than today’s text-based tutors.
Productivity
There’s already a lot of competition in this field. Microsoft is making its Copilot part of Word, Excel, Outlook, and other services. Google is doing similar things with Assistant with Bard and its productivity tools. These copilots can do a lot—such as turn a written document into a slide deck, answer questions about a spreadsheet using natural language, and summarize email threads while representing each person’s point of view.
Agents will do even more. Having one will be like having a person dedicated to helping you with various tasks and doing them independently if you want. If you have an idea for a business, an agent will help you write up a business plan, create a presentation for it, and even generate images of what your product might look like. Companies will be able to make agents available for their employees to consult directly and be part of every meeting so they can answer questions.
“If your friend just had surgery, your agent will offer to send flowers and be able to order them for you.”
Whether you work in an office or not, your agent will be able to help you in the same way that personal assistants support executives today. If your friend just had surgery, your agent will offer to send flowers and be able to order them for you. If you tell it you’d like to catch up with your old college roommate, it will work with their agent to find a time to get together, and just before you arrive, it will remind you that their oldest child just started college at the local university.
Entertainment and shopping
Already, AI can help you pick out a new TV and recommend movies, books, shows, and podcasts. Likewise, a company I’ve invested in, recently launched Pix, which lets you ask questions (“Which Robert Redford movies would I like and where can I watch them?”) and then makes recommendations based on what you’ve liked in the past. Spotify has an AI-powered DJ that not only plays songs based on your preferences but talks to you and can even call you by name.
Agents won’t simply make recommendations; they’ll help you act on them. If you want to buy a camera, you’ll have your agent read all the reviews for you, summarize them, make a recommendation, and place an order for it once you’ve made a decision. If you tell your agent that you want to watch Star Wars, it will know whether you’re subscribed to the right streaming service, and if you aren’t, it will offer to sign you up. And if you don’t know what you’re in the mood for, it will make customized suggestions and then figure out how to play the movie or show you choose.
You’ll also be able to get news and entertainment that’s been tailored to your interests. CurioAI, which creates a custom podcast on any subject you ask about, is a glimpse of what’s coming.
A shock wave in the tech industry
In short, agents will be able to help with virtually any activity and any area of life. The ramifications for the software business and for society will be profound.
In the computing industry, we talk about platforms—the technologies that apps and services are built on. Android, iOS, and Windows are all platforms. Agents will be the next platform.
“To create a new app or service, you’ll just tell your agent what you want.”
To create a new app or service, you won’t need to know how to write code or do graphic design. You’ll just tell your agent what you want. It will be able to write the code, design the look and feel of the app, create a logo, and publish the app to an online store. OpenAI’s launch of GPTs this week offers a glimpse into the future where non-developers can easily create and share their own assistants.
Agents will affect how we use software as well as how it’s written. They’ll replace search sites because they’ll be better at finding information and summarizing it for you. They’ll replace many e-commerce sites because they’ll find the best price for you and won’t be restricted to just a few vendors. They’ll replace word processors, spreadsheets, and other productivity apps. Businesses that are separate today—search advertising, social networking with advertising, shopping, productivity software—will become one business.
I don’t think any single company will dominate the agents business–there will be many different AI engines available. Today, agents are embedded in other software like word processors and spreadsheets, but eventually they’ll operate on their own. Although some agents will be free to use (and supported by ads), I think you’ll pay for most of them, which means companies will have an incentive to make agents work on your behalf and not an advertiser’s. If the number of companies that have started working on AI just this year is any indication, there will be an exceptional amount of competition, which will make agents very inexpensive.
But before the sophisticated agents I’m describing become a reality, we need to confront a number of questions about the technology and how we’ll use it. I’ve written before about the issues that AI raises, so I’ll focus specifically on agents here.
The technical challenges
Nobody has figured out yet what the data structure for an agent will look like. To create personal agents, we need a new type of database that can capture all the nuances of your interests and relationships and quickly recall the information while maintaining your privacy. We are already seeing new ways of storing information, such as vector databases, that may be better for storing data generated by machine learning models.
Another open question is about how many agents people will interact with. Will your personal agent be separate from your therapist agent and your math tutor? If so, when will you want them to work with each other and when should they stay in their lanes?
“If your agent needs to check in with you, it will speak to you or show up on your phone.”
How will you interact with your agent? Companies are exploring various options including apps, glasses, pendants, pins, and even holograms. All of these are possibilities, but I think the first big breakthrough in human-agent interaction will be earbuds. If your agent needs to check in with you, it will speak to you or show up on your phone. (“Your flight is delayed. Do you want to wait, or can I help rebook it?”) If you want, it will monitor sound coming into your ear and enhance it by blocking out background noise, amplifying speech that’s hard to hear, or making it easier to understand someone who’s speaking with a heavy accent.
There are other challenges too. There isn’t yet a standard protocol that will allow agents to talk to each other. The cost needs to come down so agents are affordable for everyone. It needs to be easier to prompt the agent in a way that will give you the right answer. We need to prevent hallucinations, especially in areas like health where accuracy is super-important, and make sure that agents don’t harm people as a result of their biases. And we don’t want agents to be able to do things they’re not supposed to. (Although I worry less about rogue agents than about human criminals using agents for malign purposes.)
Privacy and other big questions
As all of this comes together, the issues of online privacy and security will become even more urgent than they already are. You’ll want to be able to decide what information the agent has access to, so you’re confident that your data is shared with only people and companies you choose.
But who owns the data you share with your agent, and how do you ensure that it’s being used appropriately? No one wants to start getting ads related to something they told their therapist agent. Can law enforcement use your agent as evidence against you? When will your agent refuse to do something that could be harmful to you or someone else? Who picks the values that are built into agents?
There’s also the question of how much information your agent should share. Suppose you want to see a friend: If your agent talks to theirs, you don’t want it to say, “Oh, she’s seeing other friends on Tuesday and doesn’t want to include you.” And if your agent helps you write emails for work, it will need to know that it shouldn’t use personal information about you or proprietary data from a previous job.
Many of these questions are already top-of-mind for the tech industry and legislators. I recently participated in a forum on AI with other technology leaders that was organized by Sen. Chuck Schumer and attended by many U.S. senators. We shared ideas about these and other issues and talked about the need for lawmakers to adopt strong legislation.
But other issues won’t be decided by companies and governments. For example, agents could affect how we interact with friends and family. Today, you can show someone that you care about them by remembering details about their life—say, their birthday. But when they know your agent likely reminded you about it and took care of sending flowers, will it be as meaningful for them?
In the distant future, agents may even force humans to face profound questions about purpose. Imagine that agents become so good that everyone can have a high quality of life without working nearly as much. In a future like that, what would people do with their time? Would anyone still want to get an education when an agent has all the answers? Can you have a safe and thriving society when most people have a lot of free time on their hands?
But we’re a long way from that point. In the meantime, agents are coming. In the next few years, they will utterly change how we live our lives, online and off.
Source Gates Notes