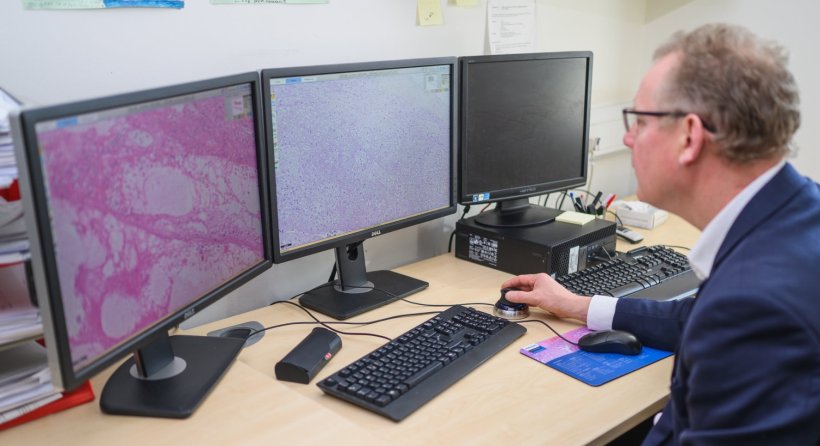
New research funded by the National Institute for Health and Care Research (NIHR) has led to the UK government approving the use of digital pathology to help speed up analysis of cancer screening samples.
This allows the benefits offered by digital pathology to be used to improve cancer screening particularly in bowel, breast, lung and cervical cancers. The use of this technology, based on research done by University Hospitals Coventry and Warwickshire (UHCW) NHS Trust and The University of Warwick’s Clinical Trials Unit, will result in faster reporting of people’s samples helping to deliver world class care. full research paper is published in the journal Histopathology.
Histopathology – the examining of cells and tissues under a microscope – is a key step in many major disease pathways, where early detection of cancer plays a crucial role in survival. Digital pathology is the use of automated slide scanners to digitise the histopathology process. Results are reported on computer workstations as opposed to a conventional microscope, enabling pathologists to report samples remote from the laboratory producing slides. This process makes sharing samples easier, helping to reduce risk of loss or damage of samples. It also mitigates the need for pathologists to be present in hospitals, as they can review the slides remotely. Digitising the slides might also allow the use of computer algorithms to help improve pathologists’ performance in the coming years.
Following a consultation by the UK National Screening Committee, the Government has now approved the use of digital pathology for analysing cancer screening samples.
The transition to using digital pathology in clinical practice […] enables many benefits to be realised, including the option to use artificial intelligence-based tools to support pathologists in their workDavid Snead
Lead Researcher Consultant Pathologist Professor David Snead, of UHCW and The University of Warwick, said: “I am delighted that digital pathology is cleared for use in cancer screening programmes. It is a big milestone to achieve and we are extremely proud that the work we have led proved so effective in making this change. The team would like to thank the pathologists, research fellows, statisticians and laboratory technicians who conducted the study and the technical support received from 3DHISTECH and Philips in providing equipment to make it happen. It was a huge task to do, but the data we produced was vital to demonstrate this technology is safe in the hands of our pathologists. UHCW can rightly claim to have led the world in the transition to using digital pathology in clinical practice. Doing so enables many benefits to be realised, including the option to use artificial intelligence-based tools to support pathologists in their work.”
Digital pathology opens up a whole new world of possibilities in diagnosis, prognosis, and prediction of diseases. Keep up-to-date with the latest research news, medical applications, and background information on digital pathology.
Professor Janet Dunn, lead for the Warwick Clinical Trials Unit, said: “It is great that the UK Government recognise the importance of this research and has approved the use of digital pathology for screening. It was a pleasure to work with Professor David Snead on this important study.”
The study was funded by the NIHR, Health Technology Assessment Programme and supported by the University of Warwick’s Clinical Trials Unit.
In total, six NHS hospitals took part in the DP study: University Hospitals Coventry and Warwickshire; The Queen’s University of Belfast; Nottingham University Hospital; Oxford John Radcliffe Hospital; United Lincolnshire Hospital and University Hospitals Birmingham. The study’s aim was to demonstrate equivalence in pathologists reporting of a sample when using digital pathology in comparison to light microscopy, the current standard. Sixteen pathologists took part in the study, four each in four specialities: Skin; Breast; GI and Renal. The pathologists reported on each anonymous sample within their speciality using both digital pathology and light microscopy. 2024 samples were used across all four specialities, a total of 16,192 reports were created during the study.
Source: University of Warwick